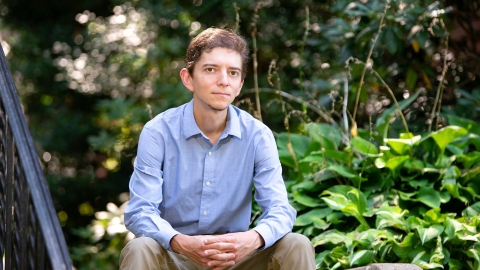
Freeman College’s Thiago Serra Receives NSF Award to Study Neural Networks
May 7, 2021
Freeman College of Management Professor Thiago Serra, business analytics. Photo by Emily Paine, Communications
When PayPal's online payments system initially launched, the company lost considerable profits due to a high fraud rate — until it developed its own fraud monitoring software named Igor.
Igor has worked effectively to stop the fraud, largely because it incorporates a neural network that "learns" new types of fraud over time. By using Igor, PayPal has brought its online fraud rate down to less than 0.5%, compared to a rate of 1.13% among other web merchants.
In addition to online shopping fraud detection, neural networks are used to support diverse services from predicting medical diagnoses to recommending content on streaming services like Amazon Prime. Now, a Bucknell University Freeman College of Management professor wants to make this technology more accessible by compressing those neural networks to fit in cell phones, smart appliances and wearable devices.
Funded by a $174,847 National Science Foundation (NSF) grant, Professor Thiago Serra, analytics & operations management, and four undergraduate students will collaborate on a two-year study aimed at developing exact neural network compression algorithms to effectively reduce their size and make them more widely available on conventional devices.
"There is a lot of money spent on this type of computing. These neural networks are typically trained and used on machines that are not affordable for everyone," Serra says. "Once you train a very large neural network to allow it to do its job well, we have techniques available to make it smaller so we can embed it in devices such as cellphones and make it less expensive to use in practice. The bulk of this work is to try to cut some pieces of the network away while not damaging too much of the predictions that these neural networks can make."
According to Serra, his team plans to only remove pieces that make no difference in the neural network's predictive responses. But just where to make those cuts without changing the network's behavior is the main question he hopes to answer through this analysis.
"I'm going to make the network smaller, if I can, but in this process, I'm not going to change how it reacts to inputs from the environment," he says. "Yet we don't really know if the way we're training these neural networks or the decisions we make about their sizes are the best ones or not. So we're going to consider if a compressed neural network, when compared to a neural network that is originally smaller but more difficult to compress, may actually work better in the long run, in terms of making better predictions."
Serra also anticipates addressing how to overcome the inherent biases found in machine learning models, which tend to be aggravated when neural networks are compressed through the most commonly used methods.
"For example, we know that most of the facial recognition systems that are used by the police and for other purposes are trained through datasets of human faces, so they can identify a suspect. However, those faces are predominantly from white and male individuals," he says. "So when you look at how effective those models are at correctly identifying white, male subjects, they're very good. But when you look at what they're not good at — for example, women and people of color — they get it wrong a lot more often. We will consider how to avoid that bias."
The NSF funding will cover summer housing and a stipend for the four students to work full time in the summers and part time during the academic year, beginning this summer. Along with attempting to develop better compression algorithms to reduce the cost and increase the accessibility of neural networks, the project will also provide a greater understanding of neural networks to the academic literature.
"We know that using these neural networks, we can come up with very powerful predictive models, but we don't really understand everything they do or why they do it so well," Serra says. "They need to be very efficient at what they do and to do it at a very accurate level, so a company or organization may need a large neural network to meet their needs, which ends up costing a lot of money. But how big should it be? We don't know. We have no clue what the right size is at present. So our research should contribute to that understanding."
The NSF funds will also be used for purchasing more machines for Bucknell's High Performance Research Computing Network (BisonNet), as well as sponsoring Bucknell students to present their findings at academic conferences.
Serra notes that the grant was largely made possible thanks to Bucknell's interdisciplinary approach to student education and faculty scholarship. The funds are provided by NSF's Directorate for Computer and Information Science and Engineering. Serra, a management professor, says Bucknell's liberal-arts-based approach offers him the freedom to pursue scholarship at the intersection of applied mathematics, computer science and management science, rather than confining his work to a particular field.