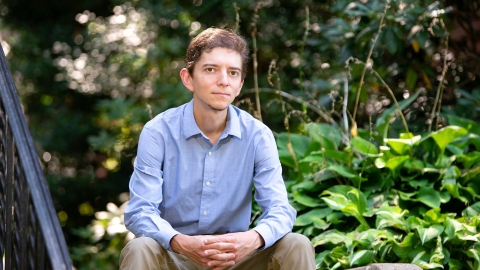
Bucknell Research: Smaller AI Models Can Be More Accurate, Fairer
July 20, 2023
Freeman College of Management Professor Thiago Serra, business analytics. Photo by Emily Paine, Communications
When it comes to neural network machine learning — recently popularized by artificial intelligence (AI) applications — a team of Bucknell University analytics researchers have found that less may be more in improving accuracy and reducing bias.
Freeman College of Management Professor Thiago Serra, analytics & operations management, and students from his analytics lab recently authored two papers showing how moderately compressing or "pruning" machine learning models called neural networks — an AI method that teaches computers to process data in a way that is inspired by the human brain — may make them more accurate and less biased. Their research was recently accepted for presentation at two prestigious AI-related conferences and may influence AI developers moving forward.
"You use data for training to come up with a neural network, and initially, everything is connected. We examined how the neural network performs with the data cut from it," Serra says. "If you start removing smaller connections that are not so important, the network actually performs better than before. That’s because the performance is more uniform when you prune it — even just a little bit."
The Bucknell researchers' initial paper showed when a moderate amount of compression is used, they can make the machine learning model less biased. They identified how to prune the neural network to reduce bias — where the neural network makes correct predictions more uniformly for different groups. Students from all four undergraduate classes collaborated with Serra on the paper, which was presented last December at NeurIPS, the leading conference about neural networks.
The team's second paper tackled neural network compression from a different angle by determining what connections to remove first to improve efficiency.
"There is very little in terms of theory for where to prune from a neural network, so we came up with our own mathematical results by extending some of my prior work," Serra says. "That work was intended to decide what neural network architectures — in terms of numbers of layers and neurons — are more expressive, and we extended it to deal with the number of connections within neural networks."
Serra presented that paper in June at the International Conference on the Integration of Constraint Programming, Artificial Intelligence, and Operations Research in Nice, France.
The research was supported, in part, by a $174,000 National Science Foundation two-year grant aimed at developing exact neural network compression algorithms to effectively reduce their size and make them more widely available on conventional devices.
"All of this has come from my own work, which I started in 2018, and from testing neural networks in my lab over the past two years," Serra says. "We can use this analysis to get neural networks to do all kinds of things, and these papers will help us leverage what they do to work better in the future."
Serra and his students will continue their neural network optimization work, particularly amid the growing interest in AI-related applications.